Cookieless Analytics – a tool comparison
A rise of data scarcity awareness in analytics has lead to a series of tools that report user behavior with a minimum of collected data. These tools try to hit a sweet-spot of not requiring any user identification (cookies) yet allowing for insights into user behavior. None of these tools would require consent under GDPR (i am not a lawyer, this is not legal advice) and they all (but one) use a time limited identification method such as a hash of ip + user agent + daily random salt.
Having worked in Digital Analytics for more than 10 years, I initially found Simple Analytics and got in touch with its creator Adrian after a discussion on “minimal data” in early 2019 (see below). Since then i’ve found 4 similar tools and tried them all out. Keep reading for more about these Tools, and get in touch with me on Twitter (@LukasGrebe) for any feedback!
Tools in alphabetical order | Pricing in the lowest traffic tier |
---|---|
Fathom | 11,67 € / month if billed yearly |
GoatCounter | 5€ / month |
Plausible | 4€ / month if billed yearly |
Simple Analytics | 9€ / month if billed yearly |
Netlify Analytics | 7,99€ / month |
Netlify Analytics is one of many server-log analytics tools. This one is the Webhosting platform Netlify providing their paying customers a simplified view into their logs
Similar tools i’ve found in the mean time, not coved here: https://analytics.angelfishstats.com/, https://friendly.is/, https://nibspace.com
Implications
A central challenge is tying multiple sessions to a single user without a cookie. No cookie means a big hole in the classic analytics data and a huge set of unknowns when it comes to attribution. These implications are a reason for enhancing or replacing 1990s era server-side log analytics with client-side javascript trackers.
However, with a time based salt in identifying a single user and thus explicitly changing IDs to not “track” individual users is new here.
Perspectives
Wich of these Tools is THE BEST ? As always: what’s best depends on how you define what’s important.
Lets take a look at these tools in regards to the different areas of digital analytics.
Define
„Why measure anything at all?“
Websites ought to be there for one reason or another. I start the Digital Analytics journey by defining what metrics would be important to look at and reveal more about how visitors are achieving what the website is set out to do!
Having visitors load your website in their browser is a functional instrument and not the reason for your website to exist. Therefore looking at Page views and Visitors isn’t quite right.
While Simple Analytics and GoatCounter offer event tracking for arbitrary data points, currently Plausible and Fathom allow configuring goals and showing conversion rates. Plausible allows selecting certain pages or events to be shown as a completed goal. Fathom stands alone (for now) in offering a monetary value.

With a simple set of customizations, the complexity of defining what needs to be measured seems trivial compared to the classic tool such as Google or Adobe analytics that allow slicing, dicing and drilling down into hundreds of dimensions, Metrics, Segments and Cohorts. But again, simplicity is the selling point of these tools:
- “Easy; if you’ve been confused by the myriad of options and flexibility of Google Analytics and Matomo that you don’t need then GoatCounter will be a breath of fresh air.”
- “without deep layers of menus”
- “stop scrolling through pages of reports and collecting gobs of personal data about your visitors, both of which you probably don’t need.”
Capture
Once we’ve defined what we want to measure and why, the second part of the analytics foundation is capturing data and making it accessible in a single-point-of-truth system. This could be your data warehouse or your analytics platform of choice.
I’ll leave out topics such as tag management, data governance, (data warehouse) ETL, solution design reference and other documentation, and let’s look at the data captured:
Theres acquisition data defaulting to Referrer data from the browser and tracking parameters in the URL’s query string. GoatCounter, Simple Analytics and Plausible overwrite the referrer with data from any of the query string parameters ref
, source
or utm_source
. Fathom and Netlify is more conservative in overwriting but Fathom does provide a JavaScript API to change the referrer in a custom page view call.
I’d like to see this expanded by all tools. Providing a second dimension to the acquisition source
, such as creative
would be powerful addition for a differentiated analysis. Overwriting the referrer works, but it removes any deeper look for example when the display ad campaign link gets posted to social media.
Beautiful tidbits: Plausible allows you to see your google search console data right within its UI. If google is the search engine of your target market, this is great as you can see what people search to find your site. Along with Simple Analytics, it also shows you the original tweet instead of t.co referrer data.
As for behavioral data - what people do on the site - all tools provide a list of about 4 Dimensions: Content or page viewed
, location or country
, device type / size
, browser
and sometimes operating system
combined with the two to three Metrics Views
, Uniques
, and for some tools bounces
and time on page
.
From an Analysts perspective the documentation can be opaque as to how these metrics are calculated and displayed. „Is that 85 phone size visits or views and what is phone size?“, “Do events and Goals extend time on page or are they non interactive?” But it honestly does not matter as the information provided by the data would not change much either way. GoatCounter created a great rundown of the different „unique“ calculations of each tool.
The different values for device type / Size are all derived from screen size:
- fathom: phone, desktop, tablet
- goatcounter: phone, large phone and small tablet, tablets and small laptops, computer monitor, computer monitor larger than HD
- plausible: desktop, laptop, tablet, mobile
- simpleanalytics: seems to be broken at point of writing.
Again two additional tidbits: Netlify Analytics shows you requests that resulted in a 404-not found error and the bandwidth used. Fathom includes free uptime monitoring with alerts via eMail, Slack, Discord, SMS or Telegram Bot if your site goes down. Neat and then you realize how important bot detection is because the uptime Pings show up as thousands of pageviews in netlify analyitcs.
The Outcome category of captured data should reflect the €s earned. Interestingly enough, none of these tools but Fathom provide an explicit tracking of (monetary) values through their goal or event Tracking! I’d love to see this added to the other tools and thanks to this Article, Plausible has added it to their issue tracker!
Report & Analyse
Given the cardinality or “size” of the data available, its one page reports all the way down. There’s not much to configure besides the date. GoatCounter, Simple Analytics and Plausible have filtered views for individual pages or goals. GoatCounter and Plausible now supports a drilldown of referal sources. All tools provide a deep-link to copy & share or bookmark for any report configuration. (Goatcounter Example)





The presentation of GoatCounter has grown on my over the past weeks of using it. It has a higher data density and more meaningful ink thanks to showing data on an hourly basis. Though i have to admit that Elon Musk is right in „Things have to look cool if you want people to care“ and frankly plausible and fathom look most professional in their presentation.
When sharing data with other parties, Fathom and Plausible provide an option to send email reports to any third party or protecting reports with a simple password(hello) and for plausible even an explicit shared link that can be revoked. These options are great for sharing data within the organization or external parties. That would be nice to see in other three Tools where you’re stuck with a single set of Login credentials, or a public link to the dashboard.
Simple Analytics appears to be alone in offering a stats API to create custom dashboards, integrating into other reporting tools, or the like.
Cross-filtering the data
I wonder why none of these tools have implemented cross-filtering the data yet! The Use-case is ideal here and would make an amazing drill down into the data set. It’s even available out of the box with Vega and other visualization frameworks. I’ve created a little demo of this:
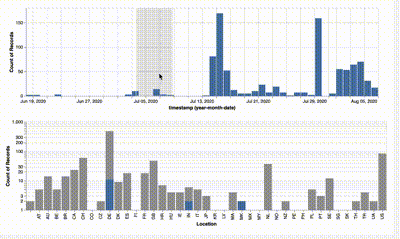
The entire dataset fits in memory and allows for a really smooth analysis. This should be expanded across all dimensions.
Test
Essential for a data-driven approach to improvement is a formal hypothesis testing when making changes. The idea is to rule out the influence of external changes on the metrics you’re watching. If the Analytics tool „understands“ testing, analyzing the impact of changes becomes a breeze. I don’t mean a fully fledged A/B testing tool that decides wich variations a visitor sees and all sorts of other things. The only requirement is to have a dimension (just like browser, or referrer) to filter usage data by and thus create a segment for visitors that experienced a specific test variation.
None of these Tools make that process easy, but it can be done with workarounds.
Fathom and GoatCounter allow creating a second tracking code for a single site, Plausible and Simple Analytics don’t but you could use event Tracking. If Segmenting for test variations (see above) isn’t an option you need at least 4 numbers: Visits to Variation and Controls, and „Success events“ for both.
Predict
Predicting any metric with these tools is going to be a manual job. You can export something from all tools, except Netlify Analytics. How useful that will be is going to be something else.
Plausible gives you a single two column export of Date and number of Visitors.
Fathom provides 7 export files:
- Browsers: “Timestamp”,“Browser”,“Version”,“Pageviews”,“Visits”
- Countries: “Timestamp”,“Country”,“Pageviews”,“Visits”
- Device Types: “Timestamp”,“Device Type”,“Pageviews”,“Visits”
- Goals: “Timestamp”,“Goal Name”,“Goal Code”,“Completions”,“Value”
- Pages: “Timestamp”,“Hostname”,“Pathname”,“Pageviews”,“Visits”
- Referrers: “Timestamp”,“URL”,“Hostname”,“Pathname”,“Pageviews”,“Visits”
- Site: “Timestamp”,“Pageviews”,“Visits”,“Avg Duration”,“Bounce Rate”
Well thats more than Plausible, but correlating anything isn’t exactly fun as the export is effectively splitting all dimensions in their own export.
Simple analytics and GoatCounter provide an export that is easier to work with. A single File with these Columns:
- Simple Analytics: added_unix, added_iso, url, referrer_raw, referrer, hostname, source, is_unique, scrolled_percentage, duration_seconds, device_width_pixels, utm_source, utm_campaign, utm_content
- GoatCounter: 1Path, Title, Event, Bot, Session, FirstVisit, Referrer, Referrer scheme, Browser, Screen size, Location, Date
Both are missing their refined / cleaned / categorized data, so, for example Browser and Browser Version will have to be parsed from the User-agent String.
So its not as easy as “grab a CSV and fire up RStudio!”, we’ve got some ETL and cleanup to do.
Context & Teamwork
Managing the complexity of the classic analytics tools is the topic of many conference talks. This could be the Analytics Team’s internal organization of managing data requests, new and changed tracking requirements or in-depth analysis task forces. Similarly all team external / context topics like documentation, data governance, training, ticket-systems for any by other teams …
Although these Topics take up a large amount of time in my day-to-day work, given the simplicity of these new tools, there isn’t much to write about. I’ll suggest creating a single documentation page of how to integrate the tracking code, your conventions for naming referrers, events, and goal value calculation. A short paragraph on accessing the data and who’s responsible et voila, you’re done.
The People behind privacy friendly analytics
All Tools but Netlify Analytics are Bootstrapped and run by one or two people. That’s great for support because they intensely care about every customer! Most my questions were answered within minutes, all within a day Even on weekends!
Tools in alphabetical order | Governing Law | Creators |
---|---|---|
Fathom | Canada | Jack Ellis and Paul Jarvis |
GoatCounter | Netherlands | Martin Tournoij |
Plausible | EU | Uku Taht and Marko Saric |
Simple Analytics | Netherlands | Adriaan van Rossum |
Netlify Analytics | USA | Netlify, Inc. |
Closing Thoughts
Practicing minimal data collection is not only honorable but a legal requirement. In a first iteration of new tools, this comes at the cost of insights and analysis depth. A Cross filtering UI would be a big improvement and should work nicely with the available data. However the available data is the limiting factor.
In theory, i think, this does not need to be the case. We need to collect not less, but different data. Ive had a few discussions about this with other Digital Analytics practitioners. Among them Till Büttner and Markus Hradsky at Web Analytics Wednesday NRW who were both inspired by the thought and lead them to embrace limitations. „Thank God for GDPR & ePrivacy“ - Till Büttner @ Measure Camp
With the tools presented here, we stick to the idea of an individual user but change the way data about that user is collected by calculating a loose and temporary ID instead of assigning an ID via a tracking cookie.
Instead we need to get away from individual Users and think more about Clusters or Groups of users with the same behavior. Define their assumed needs and measure the fulfillment of those needs for a given group.
In Practical Terms: ID a user by their behavior not an ID. The result would be a behavioral vector that identifies a cluster of users. I’m not a lawyer, so i can’t tell if this would bring back the GDRP consent request 🤔
My Choice
After working with all tools and realizing i don’t want to pay all of them inevitably for doing the same, my choice for this site landed on GoatCounter for the following reasons:
- Excellent tracker documentation
- Best Data Export. The only export in-fact capable of creating the cross-filter demo
- SaaS price
- Most “hackable” (stay tuned)
Feedback please
This is my first write up here. Please get in touch with me for any feedback at all, via twitter, email or linkedIn.
Thank you!